1. INTRODUCTION
The anatomical features of woods vary with tree species. Therefore, by observing the anatomical features of wood in cross, tangential, and radial sections of the wood under light microscope, different types of wood can be identified (IAWA Committee, 1989, 2004).
The anatomical features of softwoods typically used for wood identification are the presence or absence of intercellular resin canals, bordered pit’s lines or spiral thickening observed in axial tracheids, axial parenchyma cells, and the shape of cross field fits, rays, and aspirated pits (Choi et al., 2022; Eom and Park, 2018; Kwon et al., 2020; Lee and Bae, 2021; Lee et al., 2021a, 2021b; Nam and Kim, 2021; Park et al., 1987). Moreover, the size of wood cells or tissues can also be used to identify wood (Lee et al., 2009; Seo and Eom, 2017; Seo et al., 2014), but such data are insufficient to draw any inference.
To apply the size of wood tissues to species identification, considerable measurement data are required, which requires a huge amount of time. Also, long working hours cause both physical and mental fatigue, which in turn, may reduce measurement accuracy. To address such issues, automatic wood identification technique based on image processing is gaining popularity in recent years (Kwon et al., 2017; Lopes et al., 2021; Ravindran et al., 2021).
Quantitative wood anatomy involves quantitative analysis of anatomical features of wood and evaluation of its geographical origin and growing condition based on the anatomical features (Gebregeorgis et al., 2021; von Arx et al., 2016). To note, the anatomical structure of wood varies from tree species to species, while external environmental factors influence the size of wood cells (da Silva et al., 2021; Hwang et al., 2020; Kim et al., 2018; Seo and Eom, 2017; Seo et al., 2014). Therefore, based on this, the quantitative data can be used to identify a wood and its geographical origin. The quantitative data can also be used to control the distribution of wood of endangered species in the wood market (de Palacios et al., 2020; Lee et al., 2020; Parades-Villanueva et al., 2018; Savero et al., 2020; Yu et al., 2017).
The size of rays in a tangential section of wood is large enough to be identified under a loupe and is different for different tree species (Alves and Angyalossy-Alfonso, 2000; Burgert and Eckstein, 2001). This signifies that the axial size of the rays in the tangential section can be used as a criterion to identify wood. Furthermore, not too many studies have been done on application of ray size in wood identification.
Deep learning is a part of machine learning that enables automatic measurement, a vast amount of data acquisition and analysis through learning. Therefore, deep learning has the potential to automatically identify wood species efficiently (de Geus et al., 2021; Fabijańska et al., 2021; Fathurahman et al., 2021; Wu et al., 2021). To apply deep learning in wood identification using size of rays in tangential section, quantitative data on ray size are needed.
The present study was conducted to confirm the detectability of rays in the tangential section of conifers using mask region-based convolutional neural network (Mask R-CNN), which is an instance segmentation model among image segmentation models, and the accuracy of the instance segmentation. This work is expected to contribute to automatic wood identification means and bring improvement in the strategy of using a large amount of quantitative wood anatomical data for wood identification.
2. MATERIALS and METHODS
Softwoods such as P. jezoensis, L. gmelinii, A. nephrolepis, A. koreana, G. biloba, T. cuspidata, C. japonica, C. deodara, and P. koraiensis were selected as experimental samples (Table 1).
Cubes of length 1 cm were cut out of the experimental woods to make cross thin sections for examination under optical microscope. The cubes were softened in a mixture of glycerin and distilled water (1:4) adjusted at 60°C for 1 to 3 days. The softened cubes were cut into sections of thickness 10 to 15 μm using a small sliding microtome (GSL1, WSL), and then stained with a mixture (1:1) of astra blue (0.5%) and safranin (1%) to contrast colorations between cellulose (blue) and lignified cell walls (red). The stained thin sections were dehydrated by sequentially immersing in 30%, 50%, 70%, and 100% ethanol to minimize bubble generation while mounting with a cover glass. Euparal was used for mounting.
Digital images of cross, tangential, and radial sections were obtained using a slide scanner (Axio Scan.Z1, Zeiss, Oberkochen, Germany). Magnification of the objective lens was 20× and numerical aperture (NA) was 0.8. The images cropped to 2,048 × 2,048 pixels were used for training the model and the size per pixel was 0.220 μm × 0.220 μm.
The training course for instance segmentation requires an annotation marking the boundary of the target object area. Only tangential section images were taken, and Computer Vision Annotation Tool (CVAT) was used to annotate the rays in the tangential section. The total number of tangential images taken was 524, out of which 400 images were used for training and 124 for verification. The total number of annotations was 633, out of which 427 were used for training and 206 for verification. The annotated images were evenly selected from all tangential images to avoid overestimation of any particular image.
To quantitatively test the performance of the trained model, test images were prepared using the images that had not been used for training purpose. The boundary of the rays in the test images were marked to produce the ground truth (GT) data. The GT data were used to verify the accuracy of the annotation result by CVAT. The accuracy was evaluated as the mean average precision (mAP), which is a quantitative indicator used to select a training model for measuring the ray height.
In this study, Mask R-CNN model was used for image segmentation. Mask R-CNN is an extended version of Fast R-CNN, one of the existing object detection models, and is used to divide the boundary of an object in the detected area (He et al., 2017). Object detection is a technology to detect the location information of an object in an image and classification of objects in a detected area. On the other hand, instance segmentation is a technique to distinguish individual objects from overlapping of the same type of objects.
The Mask R-CNN model used for training is based on Python, Tensorflow, and Keras (GitHub, 2022). For transfer learning, a weight file trained using MS COCO (Microsoft Common Objects in Context) dataset was used.
The training of the model in the present study were conducted using Tensorflow 1.14.0, Keras 2.1.6, and Python 3.7.5 for segmentation of rays in the tangential section. Computers with Intel Xeon (2.2 GHz, 10 Core/20 Thread, 13.75 MB Cache), 192GB RAM, and Nvidia RTX 2080Ti were used for training.
3. RESULTS and DISCUSSION
The hyper-parameters and basic values of Mask R-CNN used in this study are as follows.
-
BACKBONE = resnet50
-
DETECTION_MIN_CONFIDENCE = 0.7
-
IMAGE_MIN_DIM = 512
-
IMAGE_MAX_DIM = 512
-
LOSS_WEIGHTS: rpn_class_loss = 1.0
-
LOSS_WEIGHTS: rpn_bbox_loss = 1.0
-
LOSS_WEIGHTS: mrcnn_class_loss = 1.0
-
LOSS_WEIGHTS: mrcnn_bbox_loss = 1.0
-
LOSS_WEIGHTS: mrcnn_mask_loss = 1.0
Among the above factors, the model was trained by varying the values of IMAGE_MIN_DIM and IMAGE_ MAX_DIM, which determine the size of the input image, and LOSS_WEIGHTS: mrcnnn_mask_loss, which greatly affects the alignment accuracy of the generated mask. The learning rate, momentum, and weight decay were 0.001, 0.9, and 0.0001, respectively. The batch size set in this study was 4, and learning was conducted for 10 to 20 epochs. The input image sizes were 512 × 512 and 1,024 × 1,024.
As a result of training under the above conditions, the highest mAP was resnet50 as a backbone, and 0.837 was found when the input image size was 1,024 × 1,024 (Table 2). The correlation analysis with GT also showed significant results, and it was confirmed that the time taken to derive the results was half that of GT. So, it was verified that the applied model could be used to automatically measure the height of rays.
When the height of the rays was high, there were rays divided into two or more rays and recognized as separate rays (Left in Fig. 1). These were called as fragmented rays in this study. To minimize the decrease in the average height of the rays due to fragmentation, image processing was done to combine the fragmented rays into one. Through image processing, a large number of fragmented rays was corrected to form combined rays (Right in Fig. 1). However, not all fragmented rays could be corrected which needs to be improved in the future.
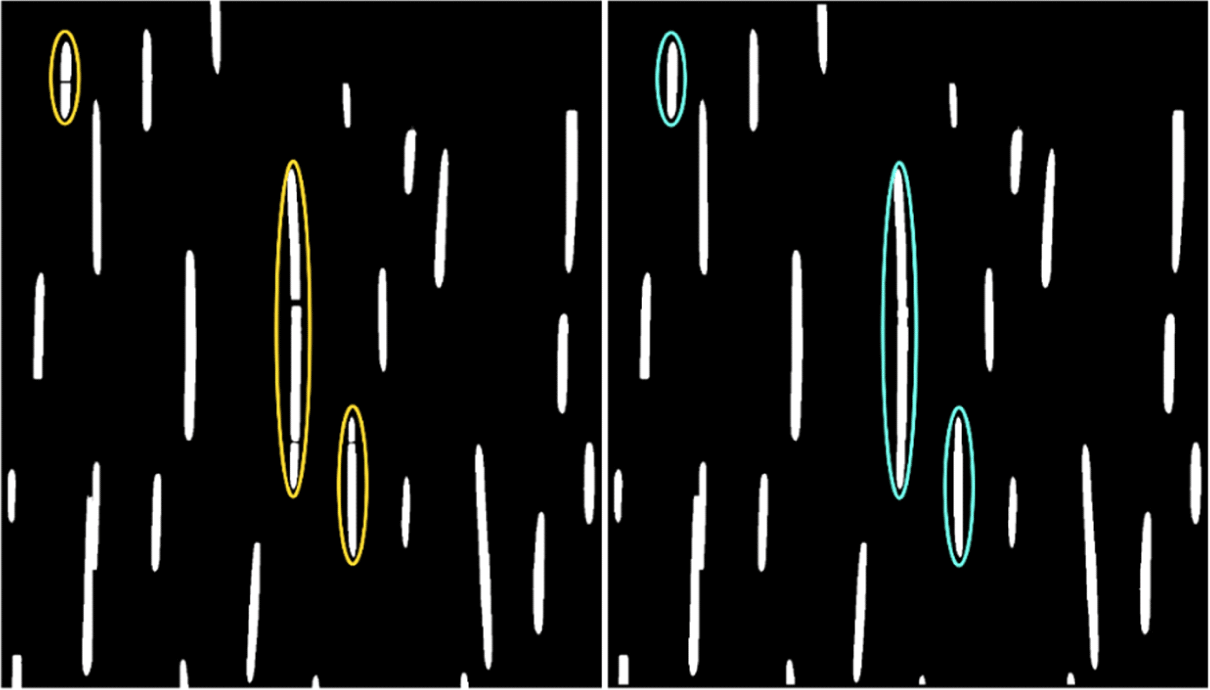
For G. biloba, T. cuspidata, and C. japonica, there was a difference of more than 100 between the numbers of rays of GT and instance segmentation (Table 3). The average height of the rays in these species did not exceed 100 μm (Fig. 2 the uppermost row). Except for P. koraiensis, the difference in the number of rays from GT and instance segmentation was relatively low. The reason for observing more differences in tree species having rays lower than 100 μm might be poor performance of Mark R-CNN for small rays. Even for tree species with relatively high rays, the mean height of the rays decreased due to fragmentation.
It was verified that kurtosis can be used as a key parameter to classify tree species (Fig. 2). Because, despite of the problem with fragmentation, there was a difference in the kurtosis of the height of the rays according to tree species except for P. koraiensis. The kurtosis of G. biloba, T. cuspidata, and C. japonica was more than 2.0, P. jezoensis, L. gmelinii, A. nephrolepis, A. koreana was approximately 1.0, and C. deodara showed negative values (Fig. 2). Only the tree species with a skewness of 2.0 or more had a mean height of rays approximately 100 μm or less.
4. CONCLUSIONS
The present study confirmed that Mask R-CNN, trained to detect rays in the tangential section, can be used to measure rays’ height. The highest mAP of the trained Mask R-CNN was 0.837. The height of the rays measured through Mask R-CNN showed a very high correlation with the results from GT, and the time to obtain the result using the model took less than half of the time required for the GT.
The frequency distribution of the ray height was verified to be unique according to the wood species. Based on these results, it was concluded that statistical values such as average values, skewness, or kurtosis of the height of rays from different wood species can be used as a basis for identification of the wood species. To apply these values, however, more improved object segmentation model and a large number of data on the height of rays are needed.
The major problems in the measurement of the height of rays using Mask R-CNN were the undetected rays and fragmentation of the rays. These two issues caused reduction in the mean ray height in each tree species. The fragmentation mostly occurred in tree species with ray height greater or equal to 100 μm. For more accuracy and reliable results, further research is necessary on improving the detection performance of the trained model and reducing the fragmentation of rays.